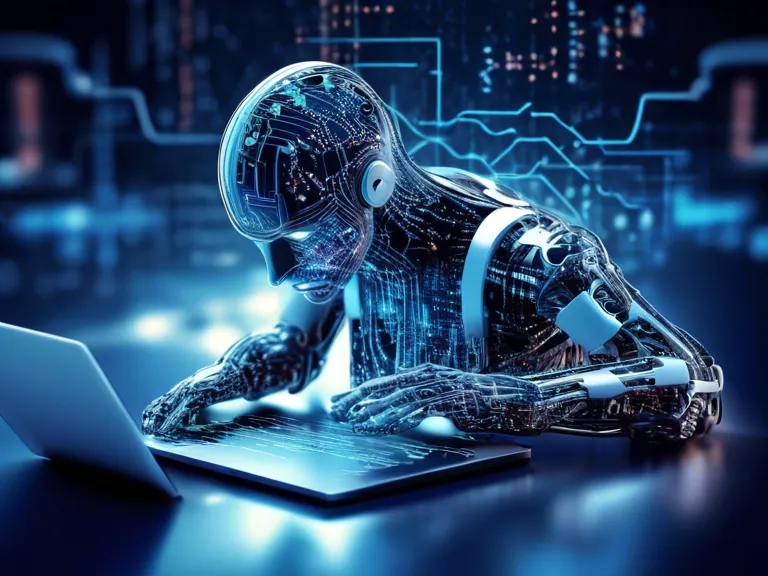
Machine learning has revolutionized various industries by enabling smart hacks and innovative solutions. With its ability to analyze vast amounts of data and learn patterns, machine learning has opened up new possibilities for businesses and individuals alike. In this article, we will explore some of the latest machine learning innovations that are driving smart hacks across different sectors.
Natural Language Processing (NLP)
Natural Language Processing is a subfield of machine learning that focuses on enabling computers to understand, interpret, and generate human language. NLP algorithms have been widely used in applications such as chatbots, sentiment analysis, and language translation. Recent advancements in NLP, such as transformer models like BERT and GPT-3, have significantly improved the accuracy and capabilities of language processing systems.
Computer Vision
Computer vision is another area where machine learning has made remarkable progress. By utilizing deep learning algorithms, computer vision systems can analyze and interpret visual data, enabling applications like facial recognition, object detection, and image classification. Innovations like convolutional neural networks (CNNs) have enhanced the performance of computer vision models, making them more accurate and efficient.
Reinforcement Learning
Reinforcement learning is a type of machine learning that involves training an agent to make decisions based on feedback from its environment. This approach has been successfully applied in various domains, including robotics, gaming, and autonomous systems. Recent advancements in reinforcement learning algorithms, such as deep Q-learning and policy gradients, have enabled agents to achieve human-level performance in complex tasks.
Generative Adversarial Networks (GANs)
Generative Adversarial Networks are a class of machine learning models that consist of two neural networks, a generator, and a discriminator, trained in a competitive manner. GANs have been used to generate realistic images, videos, and text, as well as to perform tasks like image-to-image translation and data augmentation. The ability of GANs to generate high-quality synthetic data has led to significant advancements in various creative and practical applications.
Federated Learning
Federated learning is a decentralized approach to training machine learning models across multiple devices or servers while keeping data local. This technique allows organizations to collaborate on model training without sharing sensitive data, ensuring privacy and security. Federated learning has been particularly beneficial in healthcare, finance, and other industries where data privacy is a critical concern.
Conclusion
Machine learning innovations continue to drive smart hacks and transformative solutions across different domains. From natural language processing and computer vision to reinforcement learning and generative adversarial networks, the advancements in machine learning technologies are reshaping how we interact with data and solve complex problems. By staying updated on the latest trends and leveraging these innovations, businesses and individuals can unlock new possibilities and drive meaningful impact in their respective fields.
askchrisknight.com
askchristopherknight.com
berkshirefoodjournal.com
cchirosw.com
coastalcottagesnl.com
destinationsite.com
finulldraft.com
gardenstatepaintingnj.com
impacthound.com
inneddi.com
itazurakoneko.com
judyforaz.com
localcarsnow.com
lovedayscinema.com
matiqistiqomahsambas.com
meetborofive.com
morskicovik.com
naturesrewardphotography.com
regenerativeresorts.com
restaurant-nashvilletn.com
scoopsicecreamtruck.com
simmonsmotorworks.com
trinityeco-tours.com
uncoverstudios.com
wong-multimedia.com
123exp-government.com
abozymes.com
atsheatingandair.com
bemjavim.com
caseintrend.com
cdnxbvn.com
charangolibre.com
denis-latin.com
frugal-science.com
fukuoka-kouyaren.com
hanabisou.com
herculessportcenter.com
highvoltageloja.com
hlwstoryboard.com
juderabig.com
leauxandbehold.com
melurix-next.com
moggiechicken.com
mytrendysins.com
nativeskinonline.com
ninethreestudio.com
pcmwereld.com
pichinkufibers.com
primerautobody.com
salmanhatta.com
shalevbrosh.com
sosorryrecords.com
sportsone-egy.com
wofome.com
ycadeau.com
abalawpc.com
airportpawnmiami.com
alchemycollaborative.com
blackfigdesigns.com
cubemedica.com
deliciousyarns.com
denledamtrancaocap.com
denledpanelcaocap.com
futebolrico.com
honsyuji.com
jayasupranaschool.com
jstact.com
kikichaos.com
knuckleupmartialarts.com
lavi-furniture.com
maisonsecomatiq.com
neighbors-construction.com
nspointers.com
opticiwear.com
richouli.com
soddydaisyhealthcare.com
soultalkmagazine.com
studywithpmouy.com
swordfightsandstarrynights.com
tamatur-bali.com
thepainreliefsecret.com
townhouselinens.com
vchainstore.com
vormaro.com
yukonlodgeforsale.com
airkoolny.com
an-geeke.com
bajka3.com
bertinoargenti.com
birolkitabevi.com
burikoland.com
emeraldseamless.com
floridanativecharters.com
iptvsportt.com
jupiterboutique.com
kateronline.com
kustomkoachrv.com
lademeuredufondateur.com
lucianamurta.com
luckydelaware.com
luckyindiana.com
lujannavas.com
mike4rep.com
milioshairsalon.com
movingrestaurantequipment.com
openseedvault.com
retrogradetours.com
revistaresiduos.com
roughandreadygrange.com
therusalka.com
toxnfill3.com
valleymaintenancelandscape.com
violetorlandi.com
wanpaku-golf.com
zinkdental.com
suryacctv.com
tuvannugioi.com
mandalynmusic.com
wireless-reviews.com
tussingblockwatch.com
greenteaphotography.com
jennierooney.com
vietamreview.net
kyavar.com
market-truth.com
alexandregaurier.com
omegagadget.com
banditosla.com
nursememama.com
oyunbilim.com
acme-nuclear.com
agilityimap.com
akikcombong.com
anniesmysteries.com
bflofoodie.com
brandzonestudios.com
cacemar.com
daperezlaw.com
denvyautomation.com
eugeneband.com
factory-eshop.com
florentdumas.com
hishaywireless.com
in2-signs.com
kuwekeza-holdings.com
mitaniya-ltd.com
mixfoure.com
mobilitypluspro2.com
moipravila.com
montreal-business-kit.com
mortiseandmiter.com
nextdigitaldental.com
nurdalilahputri.com
oem-phoneaccessories.com
palmbeachestepona.com
precavida.com
roscoeandetta.com
scriptsnmacros.com
sringserver.com
thecustomfairy.com
withlovefromangela.com
applebyandwood.com
auzigog.com
eac-w.com
homesbyelevation.com
nihilismforoptimists.com
slavonkandhortus.com
thekoreanpolitics.com
turningpointpt.com
val-up.com
wakansen.com
3dideation.com
achilles-fire.com
banatelhalal.com
biyografirehberi.com
bohams.com
comisiondeestudios.com
cooride-net.com
danayumul.com
ecadecom.com
edwardscornerfarmersmarket.com
ekspresif.com
ellajmae.com
ginroecooks.com
gracefueled.com
hightidekitchen.com
jeroenswolfs.com
marthalott.com
mollybroekman.com
mpthoidai.com
plumfashionpr.com
racktents.com
solzapower.com
southcoastbehavioralhealth.com
the101bali.com
thearguide.com
theartistfia.com
thefitnesswire.com
thelivelihoodproject.com
thelynndentonagency.com
wilkespoolsnspas.com
wjwatson.com
drinkganbei.com
mendenhallnews.com
nathaliemoliavko-visotzky.com
nationalinfertilityday.com
wide-aware.com
ashleymodernfurniture.com
babylonbusinessfinance.com
charliedewhirst.com
christianandmilitaryhats.com
hypnosisoneonone.com
icelandcomedyfilmfestival.com
kayelam.com
mlroadhouse.com
mumpreneursonline.com
posciesa.com
pursweets-and.com
rgparchive.com
therenegadehealthshow.com
travelingbitz.com
yutakaokada.com
22fps.com
aarondgraham.com
essentialaustin.com
femdotdot.com
harborcheese.com
innovar-env.com
mercicongo.com
oabphoto.com
pmptestprep.com
rmreflectivevest-jp.com
tempistico.com
filmintelligence.org
artisticbrit.com
avataracademyagency.com
blackteaworld.com
healthprosinrecovery.com
iancswanson.com
multiversecorpscomics.com
warrenindiana.com
growthremote.com
horizonbarcelona.com
iosdevcampcolorado.com
knoticalpr.com
kotaden.com
la-scuderia.com
nidoderatones.com
noexcuses5k.com
nolongerhome.com
oxfordcounselingcenter.com
phytacol.com
pizzaropizza.com
spotlightbd.com
tenbags.com
thetravellingwilbennetts.com
archwayintl.com
jyorganictea.com
newdadsplaybook.com
noahlemas.com
qatohost.com
redredphoto.com
rooms4nhs.com
seadragonenergy.com
spagzblox.com
toboer.com
aumantvmuseum.com
beyondausten.com
citylabstudio.com
diskonio.com
drinkcf.com
eft-dongle.com
emilymeganphotography.com
evolveathleticclub.com
godleystationvet.com
hirochanweb.com
homeonefurniture.com
ifiwasastylist.com
lacantinepopup.com
liriklagubatak.com
lo-ko.com
mensagenseatividades.com
myway-zeus.com
nevadadec.com
nokarikhabar.com
nuuuki.com
quenchpad.com
sckyrock.com
tindunghanoi.com
tradeshows-biz.com
wikimuzik.com